How can we design the optimal architecture for the next generation of AI systems capable of learning and reasoning in a manner that closely resembles human cognition?
Is deep learning alone adequate for crafting intelligent agents capable of grasping the intricate knowledge that forms the foundation of human activities? Should our emphasis expand beyond the confines of black-box number crunching and implicit representation learning towards encompassing the explicit acquisition of concepts and relationships?
Perhaps, we should explore inspiration from graph structures or even venture into the realm of biological neural networks to enhance our understanding.
Let's initiate our exploration by examining the profound impact and the burgeoning enthusiasm surrounding deep learning.
2nd Gen Data AI - Perceptual Intelligence
Deep learning has significantly advanced various domains, including computer vision, natural language processing, and speech recognition. It is often categorized as part of the second generation of AI, often referred to as Data AI, owing to its capability to discern intricate perceptual patterns when provided with substantial amounts of data.
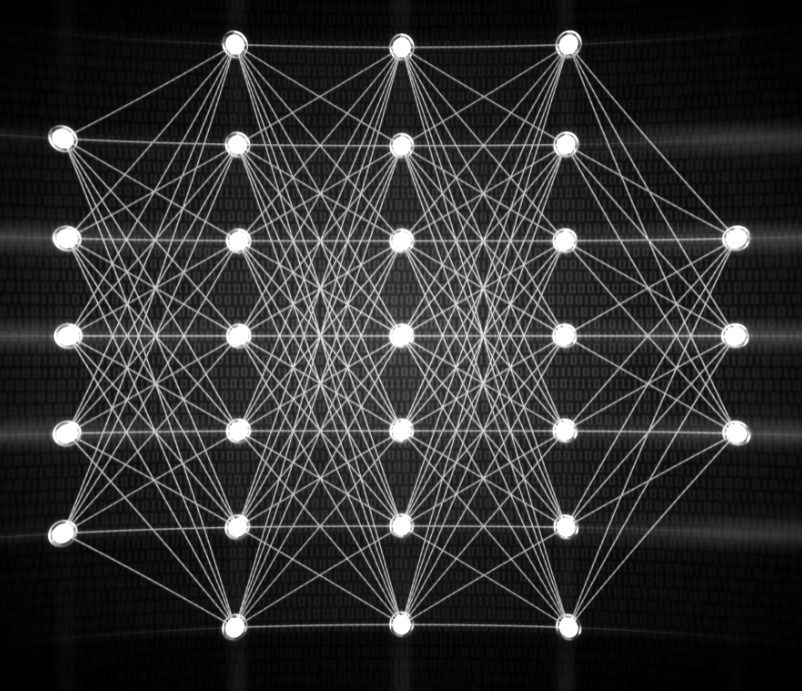
Over the past two decades (from 2000 to 2020), deep learning has undergone remarkable evolution, leaving a profound impact on numerous sectors. It has particularly transformed industries that harness the extensive data traces available on the internet, including finance, commerce, and media. Additionally, deep learning has proven invaluable in applications where the analysis of intricate perceptual patterns in areas such as vision, speech, text, or social media trails is essential.
The triumph of deep learning can be attributed to its capacity to acquire intricate non-linear feature representations that possess predictive prowess. This predictive ability emerges from the acquisition of feature representations that encapsulate significant patterns among smaller entities within any given domain. In the case of visual data, these patterns might exist among individual image pixels or patches, while in textual data, they may manifest among words.
Various iterations of artificial neural networks, including Convolutional Neural Networks (CNNs) and Recurrent Neural Networks (RNNs), employ distinct mathematical operators to capture these feature representations. More recently, Transformers have extended the capabilities of recurrent neural networks by employing multi-head attention mechanisms and positional encoding to capture pertinent patterns. This innovation has led to groundbreaking applications, exemplified by ChatGPT, and has significantly broadened the scope of AI applications.
It is essential to recognize that a significant portion of deep learning's accomplishments is confined to tasks related to Perceptual Intelligence. These include tasks such as object detection, speech recognition, and information retrieval from textual or internet content.
1st Gen Knowledge AI - Cognitive Intelligence
The real world of human activities indeed comprises intricate spatial and temporal relationships involving people and objects, essentially constituting human knowledge. To enable AI to actively engage in the real world, there is a pressing need to represent and model this human knowledge in an explicit manner. While deep learning excels at learning intricate patterns from vast amounts of data, it is not inherently designed to model the complex nuances of human knowledge in an explicit manner.
Modeling intricate world knowledge in an explicit manner is commonly referred to as Cognitive Intelligence. This form of intelligence is imperative for AI to become a valuable agent in sectors that encompass the production and movement of goods and materials.
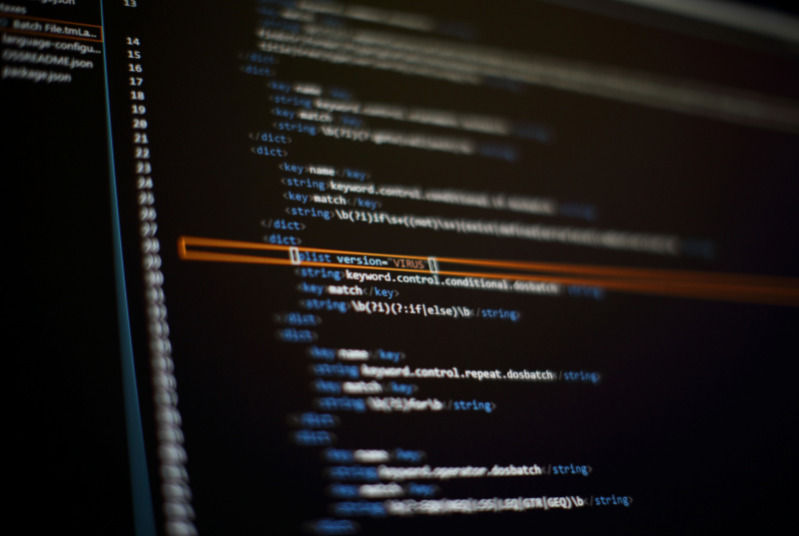
The AI agent must possess the capability to comprehend human activities not just as patterns but as knowledge, akin to how humans understand them. Numerous sectors, including manufacturing, agriculture, and autonomous driving, are poised to reap substantial benefits from the integration of Cognitive Intelligence.
Cognitive Intelligence was predominantly the domain of expert systems in the past, especially during the 1980s to the early 2000s. Expert systems excelled at modeling intricate knowledge in an explicit manner and constituted the initial wave of Knowledge AI, often referred to as the first generation.
However, these expert systems have inherent limitations in their applicability. They are not designed to learn from real-world data, which restricts their utility for intelligent agents operating in dynamic real-world environments.
3rd Gen AI: Synthesizing Perceptive and Cognitive Intelligence
Achieving a synthesis between Perceptual Intelligence and Cognitive Intelligence is indeed crucial to maximize the impact of intelligent agents in the real world. When Perception and Cognition are seamlessly integrated, it enables agents to operate effectively in a realm characterized by intricate perceptual patterns and complex human knowledge.
Creating such a synthesis necessitates the ability to both learn and reason effectively. This involves amalgamating the strengths of both the Data AI (second generation) and Knowledge AI (first generation) paradigms.
The architecture suitable for this synthesis would ideally be a hybrid model that combines the pattern recognition capabilities of deep learning, which excels in Perceptual Intelligence, with knowledge representation and reasoning techniques typically associated with expert systems or symbolic AI, which excel in Cognitive Intelligence.
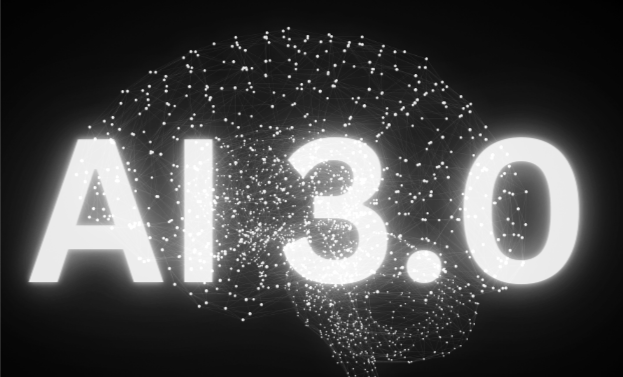
One promising approach is to develop hybrid models that integrate deep learning neural networks with symbolic reasoning systems. These models aim to bridge the gap between raw data perception and higher-level knowledge representation and reasoning. Research in this area is ongoing, and various architectures and techniques are being explored to strike the right balance between these two aspects of intelligence. This hybridization could lead to the development of more versatile and capable intelligent agents capable of understanding and acting upon complex perceptual patterns and human knowledge.
Graph Neural Networks as a possible candidate for 3rd Gen AI
Graph Neural Networks (GNNs) hold significant promise for achieving the synthesis of Perceptual Intelligence and Cognitive Intelligence, potentially paving the way for a 3rd Generation of AI. Here's why they are particularly well-suited for this:
Complex Relationship Modeling: GNNs excel at capturing intricate real-world relationships by explicitly representing entities and their connections in graph structures. This makes them highly effective in scenarios where understanding complex relationships among entities is essential.
Hierarchical Representation: GNNs have the capacity to hierarchically organize simpler entities into more complex semantic forms, mirroring the hierarchical nature of real-world systems. This enables them to represent knowledge in a structured and meaningful way, similar to how atoms form molecules and beyond.
Knowledge Representation: GNNs can explicitly represent human knowledge across various domains by structuring it within graphs. This aligns with the cognitive aspect of AI, as it allows for the explicit representation of complex knowledge.
Learning from Data: GNNs, like traditional neural networks, can learn from large volumes of data, benefiting from the wealth of information available in real-world datasets.
The combination of these characteristics positions GNNs as a strong candidate for creating a bridge between Perceptual Intelligence and Cognitive Intelligence. They have already shown remarkable results in various applications, such as recommendation systems, social network analysis, and even in natural language processing tasks.
As AI research continues to explore and develop graph-based approaches, we can anticipate further advancements that could indeed lead to the realization of a 3rd Generation of AI capable of seamlessly integrating perception and cognition for more versatile and intelligent agents.
Biological Neural Networks and Neuromorphic 3rd Gen AI
While Graph Neural Networks (GNNs) appear promising for representing real-world entities and predicting their properties, they still are rooted in the second generation of Artificial Neural Networks.
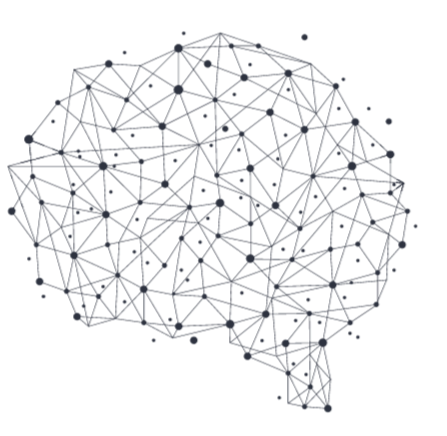
As a species, humans have demonstrated remarkable capacity for perceiving, comprehending, and innovating in their environment by harnessing abstract concepts to make sense of the tangible world. Our perception of objects goes beyond recognizing mere patterns; instead, we perceive them in the context of their conceptual attributes and their semantic interconnections with other objects and ideas.
Indeed, everything we perceive can be distilled into a conceptual framework. Basic concepts are encoded through biological neurons, while intricate ones emerge from the intricate correlations between them. Perhaps we can draw inspiration from the biological neurons' spiking or oscillatory behaviors as the foundation for advancing into the realm of the third generation of Neuromorphic AI.
For now, we'd like to leave you with these reflections. We wholeheartedly invite discussions on the topic of the third generation of AI from all perspectives, including researchers, investors, corporations, and policymakers. Your input and insights are highly valued.
Comments